Research And Development
R&D Overview
.avif)
Advancing Generative AI through Innovation
The R&D team at LightOn plays a pivotal role in advancing the field of generative AI through continuous innovation and development. Their expertise spans across creating and fine-tuning large language models (LLMs) that form the backbone of the Paradigm platform, a comprehensive AI solution designed for enterprise use. This platform simplifies the integration of generative AI into business workflows, offering both on-premise and cloud options to ensure flexibility and scalability for various business needs.
r&d publicationsRecent R&D Posts
.png)
MonoQwen-Vision, the first visual document reranker
We introduce MonoQwen2-VL-v0.1, the first visual document reranker to enhance the quality of the retrieved visual documents and take these pipelines to the next level. Reranking a small number of candidates with MonoQwen2-VL-v0.1 achieve top results on the ViDoRe leaderboard.
CTA Title
Lorem Ipsum

DuckSearch: search through Hugging Face datasets
DuckSearch is a lightweight Python library built on DuckDB, designed for efficient document search and filtering with Hugging Face datasets and standard documents.
CTA Title
Lorem Ipsum

FC-AMF-OCR Dataset : LightOn releases a 9.3 million images OCR dataset to improve real world document parsing
With over 9.3 million annotated images, this dataset offers researchers and AI developers a valuable resource for creating models adapted to real world documents.
CTA Title
Lorem Ipsum

PyLate: Flexible Training and Retrieval for ColBERT Models
We release PyLate, a new user-friendly library for training and experimenting with ColBERT models, a family of models that exhibit strong retrieval capabilities on out-of-domain data.
CTA Title
Lorem Ipsum
CTA Title
Lorem Ipsum

Training Mamba Models on AMD MI250/MI250X GPUs with Custom Kernels
In this blogpost we show how we can train a Mamba model interchangeably on both NVIDIA and AMD and we compare both training performance and convergence in both cases. This shows that our training stack is becoming more GPU-agnostic.
CTA Title
Lorem Ipsum
%20(2).avif)
Transforming LLMs into Agents for Enterprise Automation
Developing Agentic Capabilities for LLMs to automate business workflows and create smart assistants.
CTA Title
Lorem Ipsum
Explore Publications by LightOn
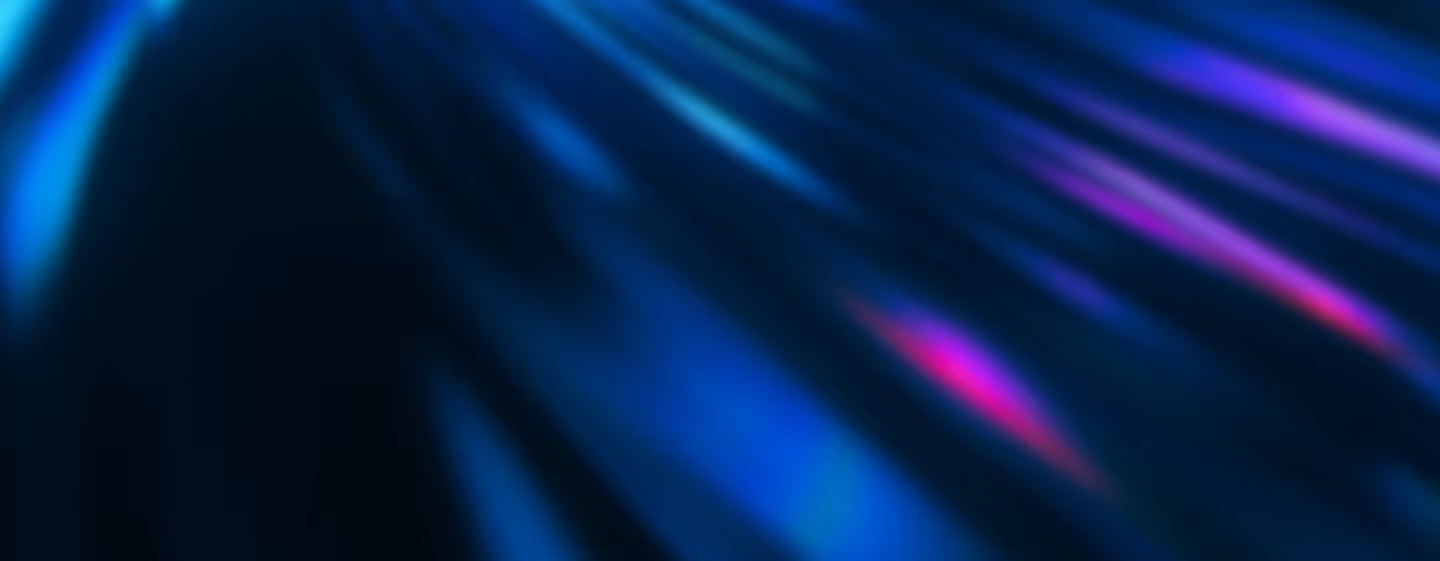
.avif)