TL;DR
Four years ago to the day, LightOn’s first Optical Processing Unit (OPU) had its first light in a Data Center showing that our technology was data center ready.
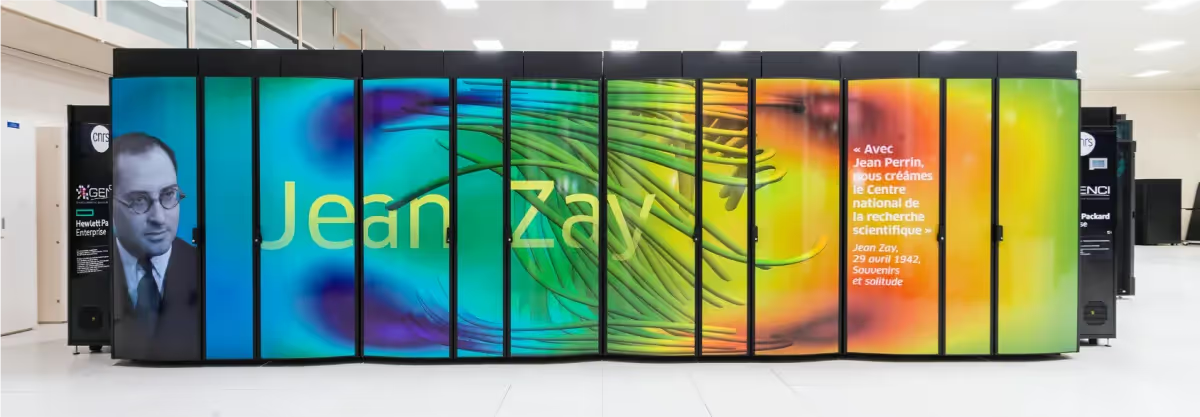
It is with immense pride and pleasure to announce that LightOn’s OPU has been installed in one of the world’s Top500 supercomputer as part of a pilot program with GENCI and IDRIS/CNRS.
The team at LightOn is immensely proud to write the future of computing in this world-first integration of a computing photonic device in an HPC infrastructure.
The press release can be found here.
Thank you GENCI and IDRIS/CNRS for making this happen!